Karhunen-Loeve Transform (KLT ) (continued)
To show that of all possible linear transforms operating on length N vectors having stationary statistics, the KTL minimizes the MSE due to truncation.
Let be some other transform having unit length basis vectors 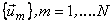
First we show heuristically that the truncation error is minimum if the basis vectors are orthogonalised using the well known Gram Schmidt procedure.
Let be the vector space spanned by the "transmitted" vectors: for and let
be the space spanned by the remaining vectors. Therefore any vector can be represented by plus vector i.e, as shown in figure below.
If is the space spanned by transmitted basis vector, then truncation error is the length of vector . Error is minimized if is to .
If only is transmitted, then MSE is the squared length of the error vector , which is clearly minimized if is orthogonal to i.e., is orthogonal to . Further orthogonalisation of the basis vectors within , or within
, does not affect MSE.
Thus we assume to be unitary i.e., has orthogonal basis vectors i.e.

Its transform coefficients are then given by

The mean square truncation error is as given by eqn ( ) is,
 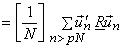
Now suppose we represent the vectors in terms of KTL basis vectors 
i.e., 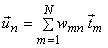
and, This follows from 
Since is unitary
(because basis vectors are of unit length)
Now from equation ( ) we have
 |
() |
and therefore can be written in terms of as, 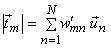 (continued in the next slide) |