|
Marginal Distributions
Let us again define two r.v., , such that the required probability space is . Let us also define and as the probability measure induced by and respectively on the space, defined by ..Now if are r.vs, such that they are defined on , where and are arbitrary Borel sets defined for and and be the joint distribution function defined for the r.v., , where is true,then represents the probability that the variable will take a value belonging to the area marked by , i.e., the probability , irrespective of the value of (remember can take any value in the entire domain of Y, i.e., ). In a similar way we can define . We can write and .
Thus the probabilities (Figure 1.9) for varying values of defines the marginal distribution of relative to the joint distribution of . In a very simple sense it means we project the mass of the joint distribution on the sub-space of the variable . Similarly we can define the probabilities (Figure 1.10) for varying values of such that it defines the marginal distribution of relative to the joint distribution of and it implies that we project the mass of the joint distribution on the sub-space of the variable .
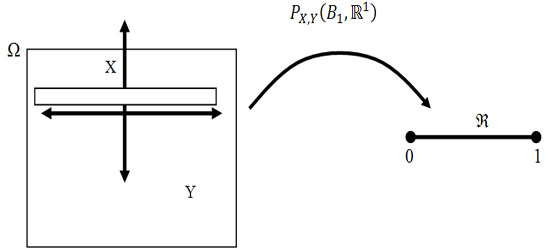 |
Figure 1.9: Illustration of |
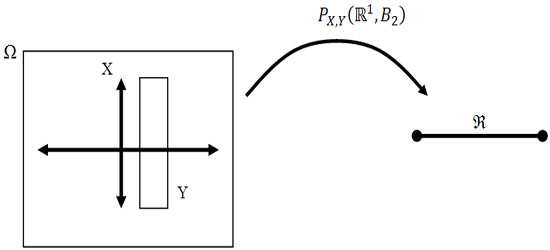 |
Figure 1.10: Illustration of
|
|